Customer indebtedness is a continuing problem - total consumer debt reported by Ofgem had reached ~£3bn by Q3 2023; and is very likely to have grown since then.
As we’ve highlighted recently, this means nearly 1m households are in significant debt to their energy supplier(s); and due to Ofgem’s method of reporting debt, the real numbers are likely much higher.
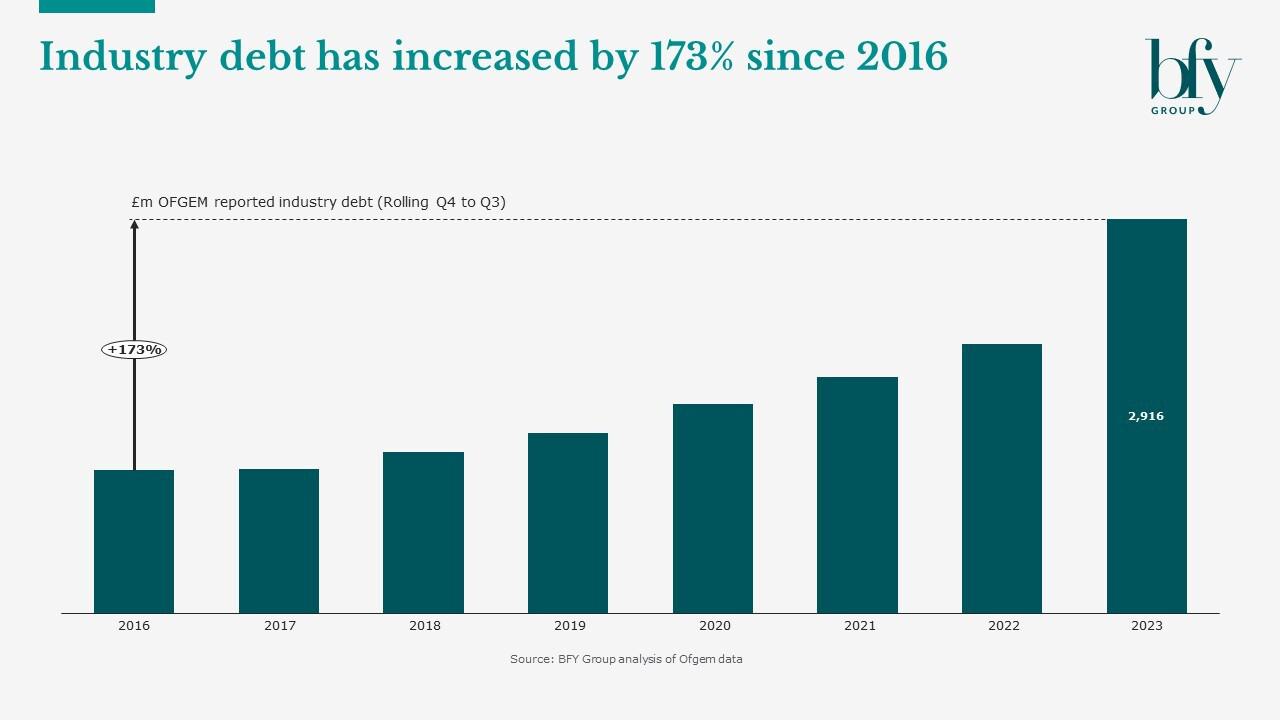
In light of this, Ofgem have announced that suppliers may now resume installing pre-payment meters, meaning suppliers can intervene where non-vulnerable customers are accruing unmanageable debt (while following robust and regulator-approved practices).
Given the recent commitment from suppliers to help households struggling with debt, more proactivity is needed to support vulnerable customers, utilising new techniques to build a comprehensive understanding of customer situations, and avoid adding further difficulty to an already challenging scenario.
Putting machine learning into practice
During a recent engagement with a large Energy retailer, we helped their Debt team to apply machine learning analysis within the customer base, identifying trends to predict (and later prevent) delinquency.
With a new insight into which customers may face financial difficulty, the supplier was able to implement pre-emptive support, through improved segmentation and tailored customer journeys.
You can read more about this approach in the case study below.
Identifying delinquency predictors for large Energy retailer
Challenge
We recently supported a large energy retailer facing an increasing Bad Debt Charge, by enhancing their capability to predict and prevent delinquency. The supplier had good visibility of customer data, but needed support in identifying the most valuable indicators of debt risk.
Working closely with our client, we helped enhance their capability to identify customers at risk of becoming indebted, and assess overall predelinquency risk.
This enabled the supplier to be proactive and offer targeted support to vulnerable customers before they might enter the collections process - reducing their Bad Debt Charge, improving operational efficiency, and helping to retain customers.
Our Approach
When given access to the supplier’s data, we applied machine learning techniques to quickly identify four predictors of delinquency. This allowed us to cut through the noise, helping our client to understand which elements of their customer data were linked closest to indebtedness.
The statistical techniques we deployed focussed on short and long-term indebtedness, enabling targeted support to be delivered.
We combined our findings into one system, providing the supplier with a measure of predelinquency risk, at both an individual account level, and for the whole customer base.
Results
This initiative was delivered as part of a wider debt management programme, which has helped our client to reduce Bad Debt Charge by £4.5m overall.
Aided by a better understanding of delinquency indicators, the supplier is now equipped to identify customers at risk of entering debt, with the capability to improve customer segmentation, and tailor customer journeys to provide proactive support.
They also have the means to perform continuous monitoring of their delinquency risk, based on the propensity of accounts to enter debt, and the expected duration of delinquency.
“Having the support to manage tactical debt resolutions has been fantastic, but the thing I am most excited about is the ability to identify and help our customers at risk of falling in to debt.”
Head of Collections
While Ofgem’s industry debt figures arouse significant concern, our debt benchmarking suggests the full view of Energy debt could be 2.5x or 3x higher than the £3bn reported. And without any plans for government support, suppliers should be focussed on offering support before debt occurs – leveraging data insights to identify the most powerful indicators of delinquency and drive proactive action.
As shown above, techniques like machine learning can help suppliers to produce actionable insights faster, informing proactive action to support customers.
If you’d like to know more about using machine learning to manage customer debt, contact John de Bono.
John de Bono
John performs various analyses to provide BFY and their clients with unique insights, which help to inform decision making and inspire exceptional performance.
View Profile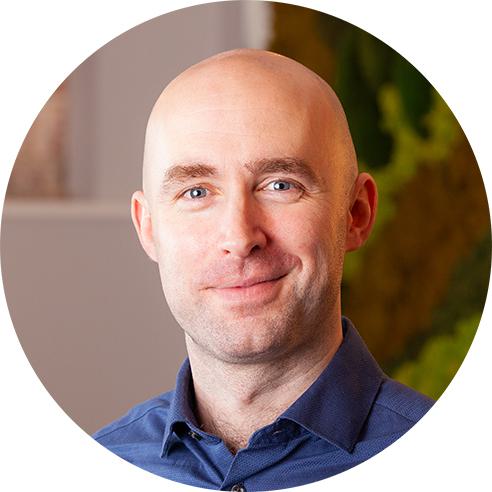